Label Images for Object Detection: The Essential Guide
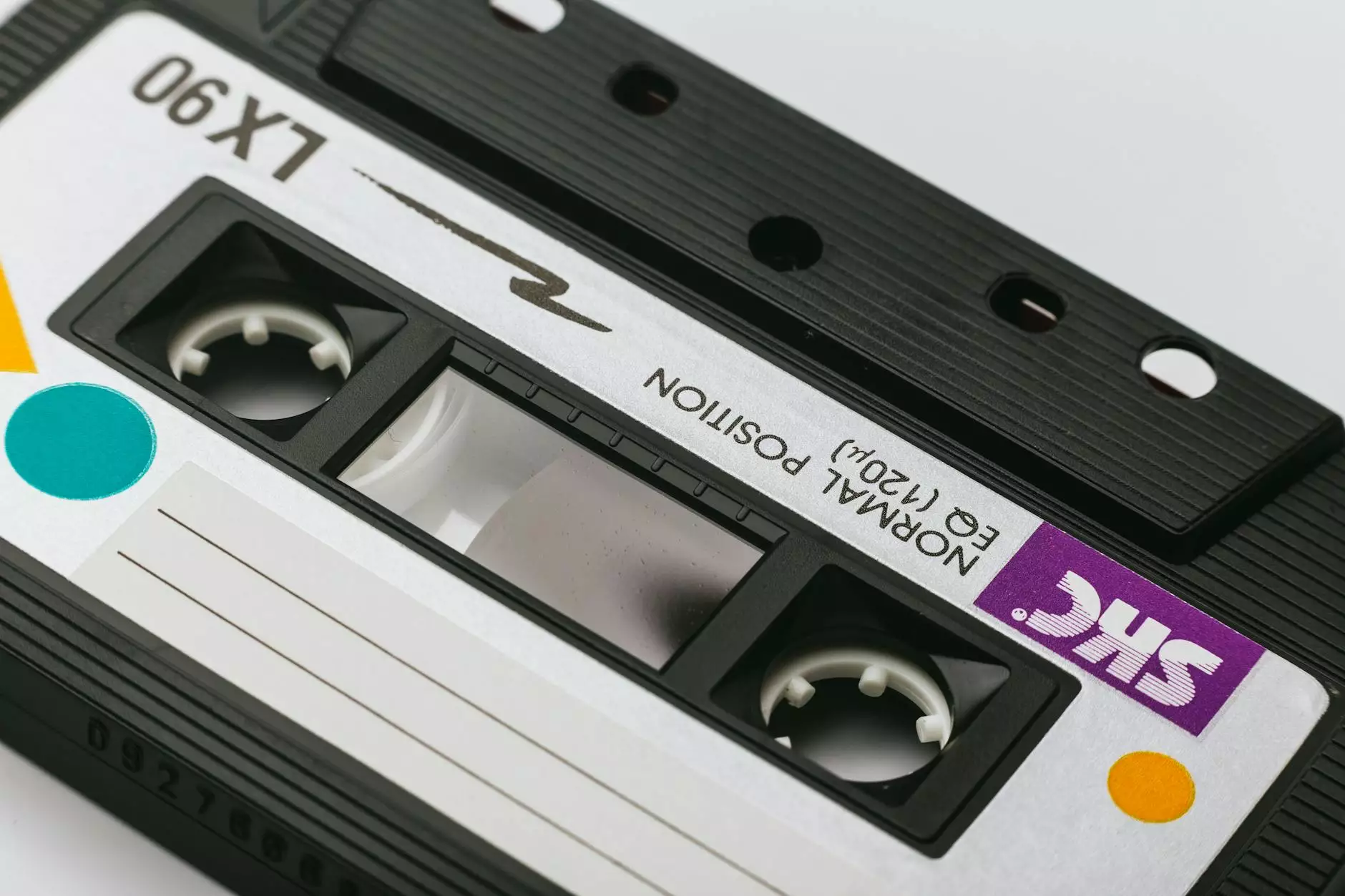
In the modern world of artificial intelligence (AI) and machine learning (ML), the process of labeling images for object detection has become increasingly significant. As businesses strive for improved accuracy in their AI models, understanding how to effectively annotate images is critical. This article delves deep into the importance of image labeling, the tools available, and how it shapes the future of object detection.
Understanding Object Detection
Object detection is a computer vision task where the goal is to detect instances of objects within an image or video. This technology is pivotal for various applications, including:
- Autonomous Vehicles: Identifying pedestrians, traffic lights, and other vehicles.
- Surveillance Systems: Monitoring and identifying unusual activities.
- Retail Analytics: Understanding customer behavior by recognizing products in stores.
At the heart of successful object detection systems lies the quality and accuracy of the image labeling process. By effectively labeling images, businesses can create reliable datasets that allow their AI models to learn with precision.
The Importance of Image Labeling
Labeling images for object detection is not just a technical necessity; it’s a strategic advantage. Here are several reasons why this process is paramount:
- Model Accuracy: Properly labeled images provide the training data necessary for ML algorithms, resulting in high accuracy rates during inference.
- Data Diversity: Well-annotated datasets showcase a wide variety of scenarios, ensuring the AI can handle different conditions and environments.
- Reduced Bias: Effective labeling helps to minimize biases in AI models by including diverse data representative of different demographics.
Types of Image Annotations for Object Detection
When organizations label images, various annotation types can be employed, each serving different purposes:
1. Bounding Box Annotations
This is the most common form of annotation, where a rectangle is drawn around an object. It is effective for detecting the location of the object without requiring precise pixel-level accuracy.
2. Polygon Annotations
For objects with irregular shapes, polygon annotations allow precision by defining a shape with multiple vertices. This is particularly useful in scenes with intricate items, like animals or architectural structures.
3. Keypoint Annotations
Used especially in spatial relationships and human pose detection, keypoints provide specific points of interest on an object.
4. Semantic Segmentation
This technique involves annotating images at the pixel level. Each pixel is labeled with a class, providing a detailed understanding of the object in context rather than just the bounding box.
Best Practices for Labeling Images for Object Detection
To ensure high-quality annotations, here are best practices to consider:
1. Consistency is Key
Ensure that all annotators are adhering to the same guidelines and standards. Consistency prevents errors and discrepancies that could mislead the model.
2. Employ Experienced Annotators
Utilize annotators who have a deep understanding of the task at hand. Experienced personnel can better understand the nuances of image labeling.
3. Implement Quality Assurance Measures
Regularly review and audit the annotations made by annotators to ensure compliance with established standards.
4. Utilize Tools Effectively
Make use of advanced data annotation tools and platforms that can streamline the labeling process while maintaining high quality. Tools that offer automation features can significantly enhance efficiency.
Choosing the Right Data Annotation Tool
With the rise of AI and ML applications, many data annotation platforms offer sophisticated tools to assist in labeling images. Here’s what to look for:
- User-Friendly Interface: A simple interface helps annotators work more efficiently.
- Collaboration Features: Tools that allow multiple users to collaborate securely can improve workflow.
- Integration Capabilities: It is vital that the platform integrates seamlessly with other systems or frameworks being used by the organization.
- Scalability: As projects grow, so should the tool capabilities. Make sure your platform can handle increased data volume as needed.
- Support and Resources: Good customer support and comprehensive documentation can enhance the user experience significantly.
Keylabs.ai: Your Partner in Data Annotation
At Keylabs.ai, we understand the critical role that labeling images for object detection plays in the success of your AI initiatives. Our data annotation platform is designed with cutting-edge features to help organizations achieve superior outcomes.
Why Choose Keylabs.ai?
Our platform stands out for various reasons:
- Advanced Automation: Reduce manual workload with our intelligent automation that assists in the annotation process.
- Robust Scalability: Whether you are a startup or an enterprise, our solutions scale to meet your needs.
- Comprehensive Support: We offer dedicated support throughout your project, ensuring you have the guidance needed for success.
- Customizable Solutions: Tailor our services to meet your project's specific needs and objectives.
Conclusion: The Future of Object Detection through Image Labeling
The path to leveraging artificial intelligence for object detection is paved with robust and precise image labeling. Investing in the right data annotation tools and adhering to best practices not only enhances the accuracy of your models but also empowers your business to stay ahead in the competitive landscape. As AI continues to evolve, the strategic handling of image labeling will remain a cornerstone of success.
Embrace the future by utilizing Keylabs.ai and ensure that your data annotation processes are streamlined and effective. Start your journey toward enhanced object detection today!
label images for object detection